In today’s technologically advanced era, the concepts of Machine Vision and Computer Vision often find themselves used interchangeably. While they share overlapping territories, they originate from different needs and address separate challenges. The distinctions, though nuanced, are significant for industry professionals, tech enthusiasts, and even consumers. So we compared for you machine vision vs computer vision!
Purpose and goal of computer vision
Machine vision vs computer vision – Although used in many types of context, let’s start with what they mean:
- Machine Vision: The primary objective of Machine Vision is to facilitate machines in performing tasks using the visual data they procure. Predominantly, it’s about enhancing the manufacturing process in applications like food industry, metal industry or any component production. This involves improving product quality, accelerating production sequences, and integrating automation. The idea is to execute a specific action based on the visual data’s interpretation. For instance, sorting defective products or piloting robotic mechanisms.
- Computer Vision: On the other hand, Computer Vision aims to simulate human-like interpretation and understanding of the visual world. The ultimate ambition is to teach machines the art of making decisions grounded on visual data, devoid of the necessity to execute physical tasks. This realm emphasizes comprehending image and video content, suitable for a wide spectrum of applications.
Application areas of machine vision and computer vision
- Machine Vision: Primarily, machine vision finds its footing in industrial terrains. Picture a bustling factory where products on assembly lines are inspected, or robotic arms are guided for precision. Here, the focus leans towards real-world, tangible applications that enhance production efficacy and quality.
- Computer Vision: This domain, conversely, spreads its wings across a broader spectrum. From the facial recognition systems in your smartphones to the object detection in advanced security systems, to the immersive realms of virtual reality and augmented reality, computer vision is the silent force that powers them. Its presence is omnipresent, both in niche industries and mainstream consumer tech.
Process Nuances of machine vision
- Machine Vision: Dive into a factory, and you’ll witness the machine vision process in its full swing. The ecosystem begins with image capture, transitioning into processing, and concludes with a specific action, like a product’s approval or rejection. Specialized hardware often aids this journey, ensuring that the image capture and analysis are top-notch.
- Computer Vision: The storyline is a tad different here. Yes, image capture and processing remain at the core. Yet, the heartbeats lie in analyzing image content. This discipline is profoundly software-centric. Algorithms, AI, and machine learning models become the heroes, making sense of the sprawling visual data.
Complexity Spectrum
- Machine Vision: The environments where machine vision thrives are usually controlled. Be it lighting, object types, or camera angles; everything is pre-defined. This controlled setting, while simplifying tasks, ensures consistent and high-quality outcomes.
- Computer Vision: Venture into the wild, and that’s where computer vision plays its game. Uncontrolled environments with unpredictable lighting, myriad angles, and diverse subject matters form the playground. Recognizing objects in a random internet snapshot or discerning patterns in bustling cityscapes, computer vision loves challenges and is also very good in video processing.
Unraveling machine learning, AI or artificial intelligence in Computer Vision solutions
As industries increasingly lean on technology for precision and efficiency, the synergy between AI and vision systems becomes unmissable. Artificial Intelligence, with its data-driven insights and predictive prowess, has amplified the capacities of both Machine and Computer Vision. But what does this amalgamation truly signify? For Machine Vision, AI transcends it from a system of fixed responses to a dynamic entity that adapts, learns, and evolves. It’s no longer just about identifying product defects; it’s about predicting them, optimizing processes, and ensuring unparalleled quality assurance. Meanwhile, Computer Vision, under the aegis of AI, has morphed into a tool that doesn’t just ‘see’ but truly ‘comprehends.’ It bridges the gap between mere visual data capture and nuanced understanding, bringing closer the dream of machines that can interpret the world as we do.
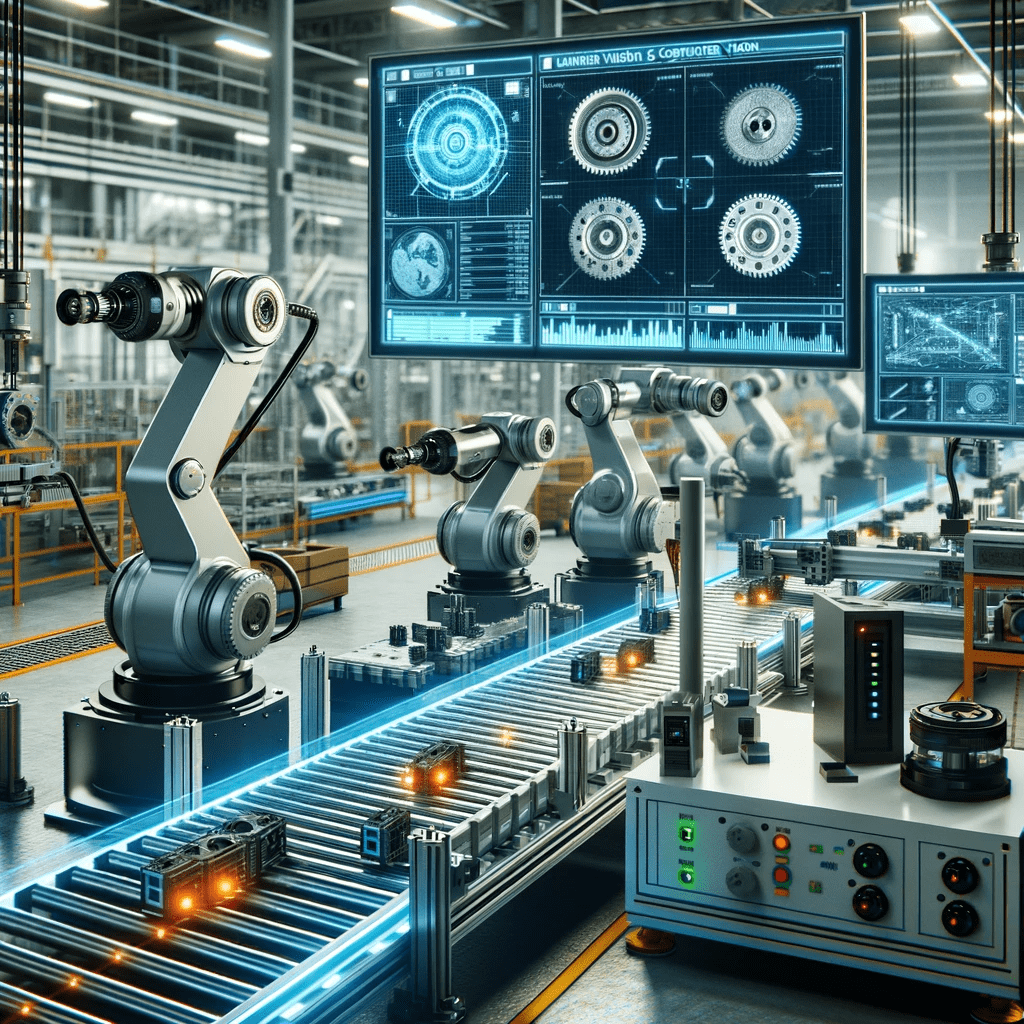
The AI-Driven Future of Vision Systems
The marriage of AI with Machine and Computer Vision is not just a technological advancement; it’s a paradigm shift. As Machine Vision systems adopt AI, industries stand to benefit from heightened efficiency and reduced error rates. On the other hand, AI-backed Computer Vision paves the way for innovations that reshape how we interact with machines, from AI-assisted medical diagnoses to smart urban planning. This fusion, promising transformative outcomes, beckons industries and innovators to invest, explore, and harness the full potential of AI-integrated vision systems. The horizon looks promising, with machines that don’t just work alongside us but understand, anticipate, and innovate with us.
In Retrospect
It’s evident that while machine vision can be perceived as a specialized subset of computer vision, focusing on industrial tasks, computer vision paints on a broader canvas. The latter seeks to infuse machines with a vision comparable to human cognition. Both are transformative in their right, steering the present and future of technology-driven ecosystems.
As we transition into a world where automation, AI, and tech-driven processes become the norm, understanding the nuances between machine vision and computer vision becomes imperative. Whether you’re an industry professional, a tech aficionado, or a curious consumer, diving into these realms offers insights into the ever-evolving technological landscape we reside in.
Conclusion
machine vision vs computer vision: In an era where visual data reigns supreme, machine vision and computer vision stand as twin pillars, each with its distinctive character. Their amalgamation and individual prowess promise a future where machines don’t just see – they understand, interpret, and act, opening new horizons for innovation.
Q: What is the difference between machine vision and computer vision?
A: Machine vision and computer vision are often used interchangeably, but there is a subtle difference. Machine vision refers to the use of computer vision specifically in industrial applications, such as quality control in manufacturing, while computer vision has a broader scope, encompassing a wide range of applications including robotics, healthcare, and augmented reality.
Q: How do machine vision and computer vision work together?
A: Both machine vision and computer vision work by using cameras and image processing algorithms to analyze and interpret visual data. They rely on image analysis, pattern recognition, and machine learning techniques to process images and extract relevant information. A big trend now is to use a vision platform or a machine vision platform to easily and quickly deploy computer vision systems.
Q: What are the key differences between computer vision and machine vision technologies?
A: The key difference lies in their respective applications. Computer vision technology is used in diverse fields such as object recognition, image analysis, and autonomous vehicles, whereas machine vision technology focuses on inspection, measurement, and quality control in industrial settings.
Q: What are the main use cases for machine vision applications?
A: Machine vision is commonly used in manufacturing for tasks such as defect detection, assembly verification, barcode reading, and robotic guidance. It can also be applied in industries like pharmaceuticals, automotive, electronics, and packaging.
Q: How does computer vision differ from machine vision in terms of its use and applications?
A: Computer vision seeks to enable computers to “see” and understand the visual world in a manner similar to humans, while machine vision is used for specific industrial applications, aiming to enhance production processes, ensure product quality, and automate tasks on the production line.
Q: What are the new and emerging applications of computer vision and machine vision technologies?
A: Both computer vision and machine vision are finding new applications in areas such as healthcare pictures, agriculture, retail, security surveillance, and augmented reality. Advanced computer vision techniques are also being applied in fields like image analysis, autonomous vehicles, and smart infrastructure.
Q: Can you explain the difference between machine vision and computer vision in the context of machine learning?
A: While both machine vision and computer vision utilize algorithms for image processing and analysis, machine vision focuses on training models, mostly deep learning, to perform specific tasks related to industrial automation and quality control, whereas computer vision explores a wider range of applications, including image recognition, object detection, and semantic segmentation.
Q: How do computer vision and machine vision technologies contribute to autonomous systems?
A: Computer vision and machine vision are integral components of autonomous systems such as self-driving cars, drones, and robotic machinery. They enable these systems to perceive their environments, make informed decisions, and navigate autonomously by processing visual inputs and detecting objects, obstacles, and spatial information.
Q: What is the role of image processing and frame grabbers in machine vision solutions?
A: Image processing and frame grabbers are essential components of machine vision systems. Processing algorithms analyze visual data to extract meaningful information, while frame grabbers capture digital images from cameras and transfer them to the processing unit for further analysis and decision-making.
Q: How does computer vision work together with machine learning in developing vision solutions?
A: Computer vision and machine learning intersect in the development of vision solutions, where machine learning techniques are applied to train models for tasks such as object recognition, scene understanding, and image classification, thereby enhancing the capabilities of computer vision systems in diverse real-world applications.